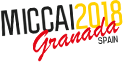
Members of the BioMedIA Group will present some of their latest research results as peer reviewed full papers at the 21st International Conference on Medical Image Computing and Computer Assisted Intervention (MICCAI).
Benjamin Hou, Nina Miolane, Bishesh Khanal, Matthew C.H. Lee, Amir Alansary, Steven McDonagh, Jo V. Hajnal, Daniel Rueckert, Ben Glocker, Bernhard Kainz
Computing CNN Loss and Gradients for Pose Estimation with Riemannian Geometry
Amir Alansary, Loic Le Folgoc, Ghislain Vaillant, Ozan Oktay, Yuanwei Li, Wenjia Bai, Jonathan Passerat-Palmbach, Ricardo Guerrero, Konstantinos Kamnitsas, Benjamin Hou, Steven McDonagh, Ben Glocker, Bernhard Kainz, Daniel Rueckert
Automatic View Planning with Multi-scale Deep Reinforcement Learning Agents
Daniel Castro, Ben Glocker
Nonparametric Density Flows for MRI Intensity Normalisation
Robert Robinson, Ozan Oktay, Wenjia Bai, Vanya Valindria, Mihir Sanghvi, Nay Aung, José Paiva, Filip Zemrak, Kenneth Fung, Elena Lukaschuk, Aaron Lee, Valentina Carapella, Young Jin Kim, Bernhard Kainz, Stefan Piechnik, Stefan Neubauer, Steffen Petersen, Chris Page, Daniel Rueckert, Ben Glocker
Real-time Prediction of Segmentation Quality
Yuanwei Li, Bishesh Khanal, Benjamin Hou, Amir Alansary, Juan Cerrolaza, Matthew Sinclair, Jacqueline Matthew, Chandni Gupta, Caroline Knight, Bernhard Kainz, Daniel Rueckert
Standard Plane Detection in 3D Fetal Ultrasound Using an Iterative Transformation Network
Juan Cerrolaza, Yuanwei Li, Carlo Biffi, Alberto Gomez, Matthew Sinclair, Jacqueline Matthew, Caroline Knight, Bernhard Kainz, Daniel Rueckert
3D Fetal Skull Reconstruction from 2DUS via Deep Conditional Generative Networks
Yuanwei Li, Amir Alansary, Juan Cerrolaza, Bishesh Khanal, Matthew Sinclair, Jacqueline Matthew, Chandni Gupta, Caroline Knight, Bernhard Kainz, Daniel Rueckert
Fast Multiple Landmark Localisation Using a Patch-based Iterative Network
Ilkay Oksuz, Bram Ruijsink, Esther Puyol-Anton, Aurelien Bustin, Gastao Cruz, Claudia Prieto, Daniel Rueckert, Julia A. Schnabel, Andrew P. King.
Deep Learning using K-space Based Data Augmentation for Automated Cardiac MR Motion Artefact Detection
Wenjia Bai, Hideaki Suzuki, Chen Qin, Giacomo Tarroni, Ozan Oktay, Paul M. Matthews, Daniel Rueckert
Recurrent Neural Networks for Aortic Image Sequence Segmentation with Sparse Annotations
Jo Schlemper, Ozan Oktay, Wenjia Bai, Daniel C. Castro, Jinming Duan, Chen Qin, Jo Hajnal, Daniel Rueckert
Cardiac MR Segmentation from Undersampled k-space using Deep Latent Representation Learning
Jo Schlemper, Guang Yang, Pedro Ferreira, Andrew Scott, Laura-Ann McGill, Zohya Khalique, Margarita Gorodezky, Malte Roehl, Jennifer Keegan, Dudley Pennell, David Firmin, Daniel Rueckert
Stochastic Deep Compressive Sensing for the Reconstruction of Diffusion Tensor Cardiac MRI
Carlo Biffi, Ozan Oktay, Giacomo Tarroni, Wenjia Bai, Antonio De Marvao, Georgia Doumou, Martin Rajchl, Reem Bedair, Sanjay Prasad, Stuart Cook, Declan O’Regan, Daniel Rueckert
Learning Interpretable Anatomical Features Through Deep Generative Models: Application to Cardiac Remodeling
Jinming Duan, Jo Schlemper, Wenjia Bai, Timothy J W Dawes, Ghalib Bello, Georgia Doumoud, Antonio De Marvao, Declan O’Regan, Daniel Rueckert
Deep nested level sets: Fully automated segmentation of cardiac MR images in patients with pulmonary hypertension
Giacomo Tarroni, Ozan Oktay, Matthew Sinclair, Wenjia Bai, Andreas Schuh, Hideaki Suzuki, Antonio de Marvao, Declan O’Regan, Stuart Cook, Daniel Rueckert
A Comprehensive Approach for Learning-based Fully-Automated Inter-slice Motion Correction for Short-Axis Cine Cardiac MR Image Stacks
Chen Qin, Wenjia Bai, Jo Schlemper, Steffen E. Petersen, Stefan K. Piechnik, Stefan Neubauer, Daniel Rueckert
Joint Learning of Motion Estimation and Segmentation for Cardiac MR Image Sequences
Maximilian Seitzer, Guang Yang, Jo Schlemper, Ozan Oktay, Tobias Wuerfl, Vincent Christlein, Tom Wong, Raad Mohiaddin, David Firmin, Jennifer Keegan, Daniel Rueckert, Andreas Maier
Adversarial and Perceptual Refinement for Compressed Sensing MRI Reconstruction