Moving objects cause motion artefacts when their enclosing volume is acquired as a stack of image slices.
We present a fast multi-GPU accelerated implementation of slice-to-volume registration based super-resolution reconstruction with automatic outlier rejection and intensity bias correction. We introduce a novel fully automatic selection procedure for the image stack with the least motion, which will serve as an initial registration template. We fully evaluate our method and its high dimensional parameter space. Testing is done using artificially motion corrupted phantom datasets and using real world scenarios for the reconstruction of foetal organs from in-utero prenatal Magnetic Resonance Imaging and for the motion compensation of freehand compound Ultrasound data. On average we achieve a speed-up of more than 40x compared to a single CPU system, and another 1.70x for each additional GPU, while maintaining the same image quality as if calculated on a CPU. Our framework is qualitatively more accurate and on average 10x times faster than currently available state-of-the-art multi-core methods.
The source code for this approach is open source and publicly available for download on github.
GPU accelerated Motion compensation for medical images
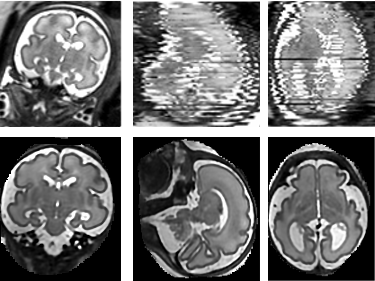